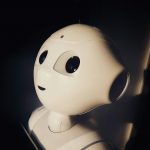
Access to mental health support is not equally distributed (Centre for Mental Health, 2020). Despite recent government commitments to improve the accessibility of mental health services, differences still exist in certain population groups’ “ability to seek” and “ability to reach” services (Lowther-Payne et al., 2023). Key barriers include experiences of – or anticipating experiences of – stigma, as well as trust in mental health professionals (Lowther-Payne et al., 2023).
In a recent paper, Habicht and colleagues (2024) suggest that there is strong evidence that digital tools may help overcome inequalities in treatment access. The authors were mainly referring to Limbic, a personalised artificial intelligence (AI) enabled chatbot solution for self-referral. This personalised self-referral chatbot is visible to any individual who visits the service’s website and collects information required by the NHS Talking Therapies services as well as clinical information such as the PHQ-9 and GAD-7. All data are attached to a referral record within the NHS Talking Therapies services electronic health record – “to support the clinician providing high-quality, high-efficiency clinical assessment”.
So are chatbots the answer to inequalities in treatment access? Within this blog we take a closer look at the evidence behind Habicht and colleagues’ claim and ask where this leaves us going forward.
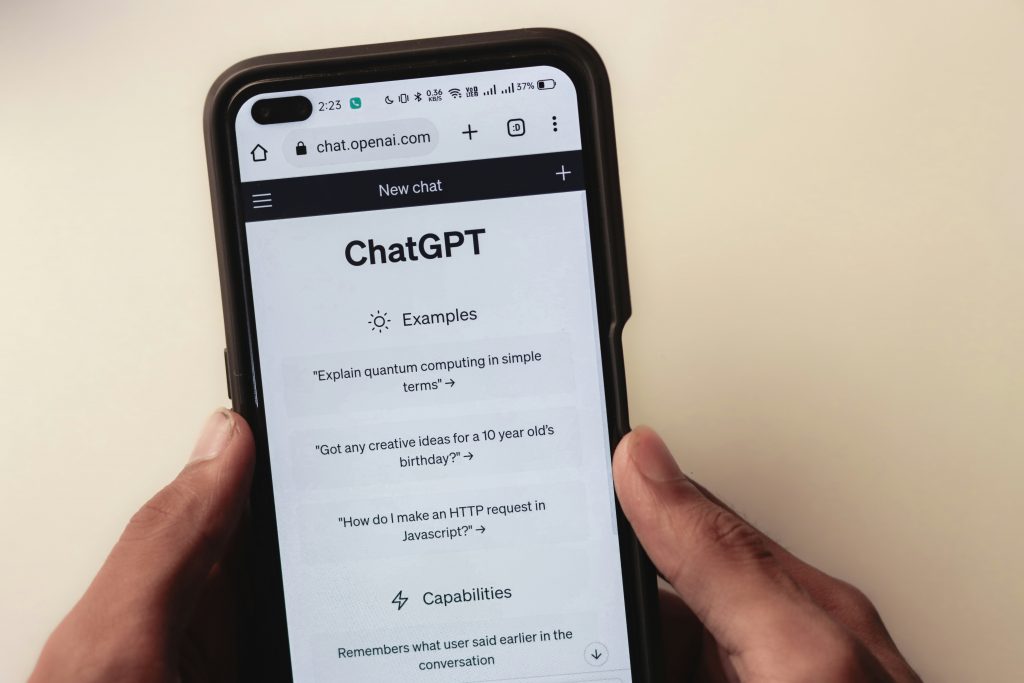
Are chatbots the answer to reducing inequalities in mental health treatment access? Habicht and colleagues (2024) suggest they are.
Methods
The authors conducted an observational real-world study using data from 129,400 patients referred to 28 different NHS Talking Therapies services across England. Fourteen of these services implemented the self-referral chatbot and these were matched with 14 services who did not. The authors paid considerable attention to this matching and only included control services that used an online form (rather than calling in to a service) as this was considered the closest referral option to the chatbot. Other considerations included:
- Number of referrals at baseline
- Recovery rates
- Wait times.
Analysis investigated 3 months before adoption of the chatbot and 3 months after launch, and primarily focused on an increase in the number of referrals. To disentangle the contribution of the AI and the general usability of the self-referral chatbot, a separate randomised controlled between-subjects study with three arms directly compared the personalised chatbot with a standard webform and an interactive (but not AI-enabled) chatbot. To explore any potential mechanisms driving findings, the authors also employed a machine learning approach – namely Natural Language Processing (NLP) to analyse feedback given by patients who used the personalised self-referral chatbot.
Results
Services that used the digital solution identified increased referrals. More specifically, those services which used the personalised self-referral chatbot saw an increase from 30,690 to 36,070 referrals (15%). Matched NHS Talking Therapies services with a similar number of total referrals in the pre-implementation period saw a smaller increase from 30,425 to 32,240 referrals (6%).
Perhaps of greater significance, a larger increase was identified for gender and ethnic minority groups:
- Referrals for individuals who identified as nonbinary increased by 179% in services which utilised the chatbot; compared to a 5% decrease in matched control services.
- The number of referrals from ethnic minority groups was also significantly higher when compared to White individuals: a 39% increase for Asian and Asian British Groups was observed, alongside a 40% increase for Black and Black British individuals in services using the chatbot. This was significantly higher than the 8% and 4% seen in control services.
Average wait times were also compared to address concerns that increased referrals may lead to longer wait times and worse outcomes. This revealed no significant differences in wait times between pre- and post-implementation periods of the services that used the chatbot and those that did not. Analysis of the number of clinical assessments suggest that the chatbot did not have a negative impact on the number of assessments conducted.
So why is the chatbot increasing referrals? And why is this increase larger for some minority groups?
According to the authors, the usage of the AI “for the personalization of empathetic responses and the customization of clinical questions have a critical role in improving user experience with digital self-referral formats”. Analysis of free text provided at the end of the referral process (n = 42,332) found nine distinct themes:
- Four were positive:
- ‘Convenient’,
- ‘provided hope’,
- ‘self-realization’, and
- ‘human-free’
- Two were neutral:
- ‘Needed specific support’ and
- ‘other neutral feedback’
- Three were negative:
- ‘Expected support sooner’,
- ‘wanted urgent support’ and
- ‘other negative feedback’.
Individuals from gender minority groups mentioned the absence of human involvement more frequently than females and males. Individuals from Asian and Black ethnic groups mentioned self-realization about the need for treatment more than White individuals.
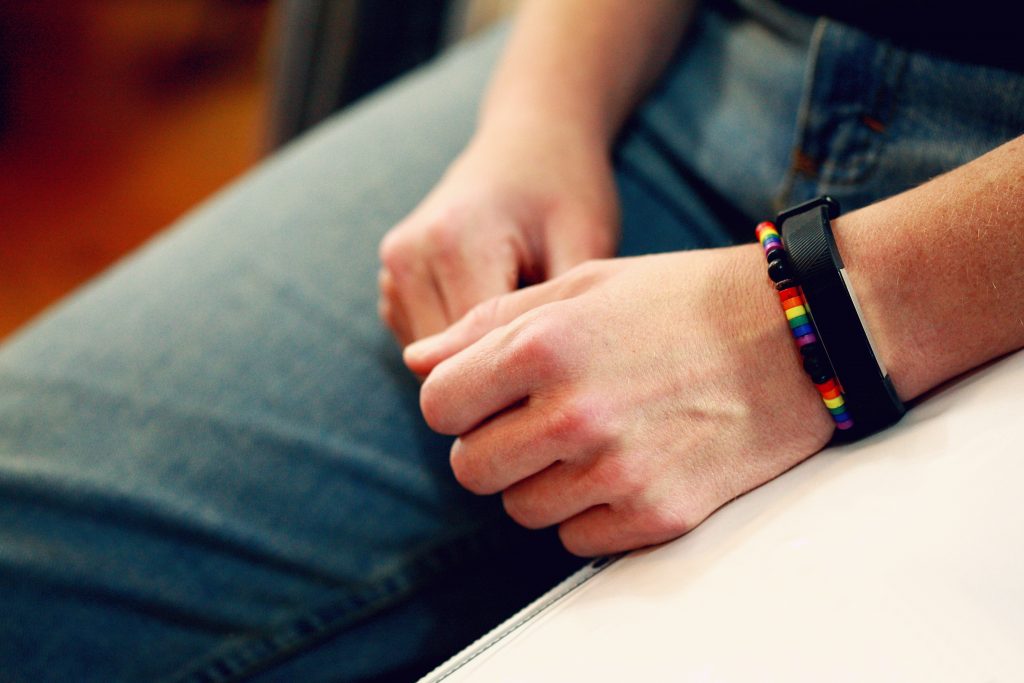
Services that used the chatbot identified increased referrals (15% increase versus 6% increase in control services). This increase was more pronounced within minority groups.
Conclusions
Findings strongly point toward the fact that personalised AI-enabled chatbots can increase self-referrals to mental health services without negatively impacting wait times or clinical assessments. Critically, the increase in self-referrals is more pronounced in minority groups, suggesting that this technology may help close the accessibility gap to mental health treatment. The fact that ‘human-free’ was identified as a positive by participants suggests that reduced stigma may be an important mechanism.
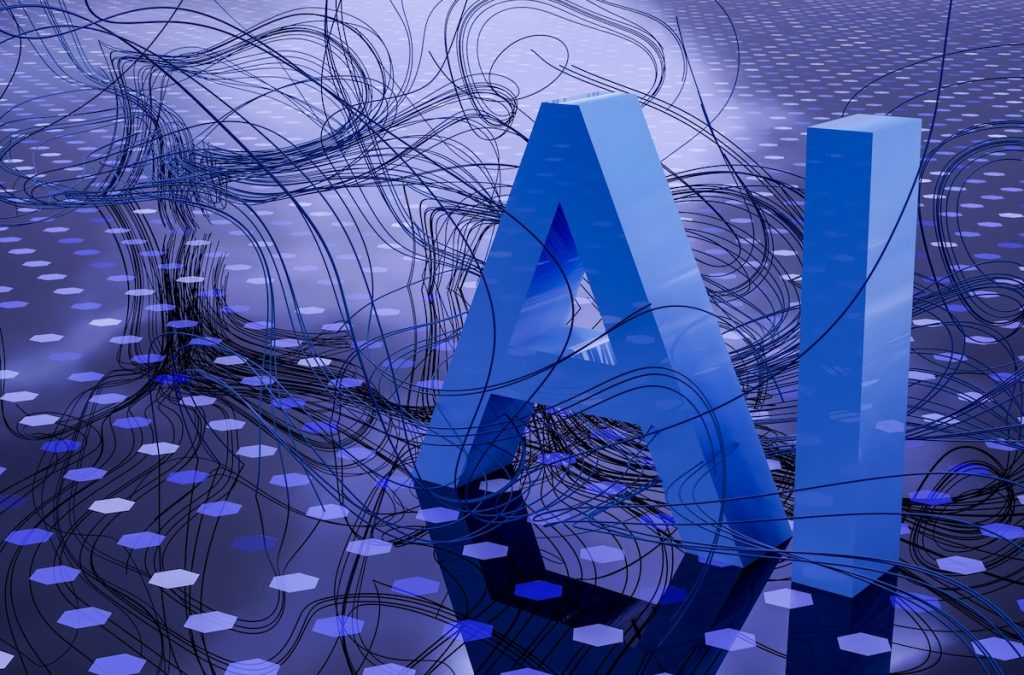
The fact that ‘human-free’ was identified as a positive by participants suggests that reduced stigma may be one reason why we see improvement in the diversity of access.
Strengths and limitations
This is a well-considered study, with convincing findings. The authors have given considerable thought to how services should be matched and devised a series of parallel analyses to control for confounders and disentangle possible mechanisms, which increases the reliability of the findings. At the same time, this drive toward robustness has the potential to downplay some of the complexities at play when considering inequalities to treatment access.
This is perhaps best seen in the NLP topic classification and discussion of ‘potential mechanisms’. According to Leesen et al. (2019), qualitative researchers may find NLP helpful to support their analysis in two ways:
- First, if we perform NLP after traditional analysis, it permits us to evaluate the probable accuracy of codes created.
- Second, researchers can perform NLP prior to open coding and use NLP results to guide creation of the codes. In this instance, it is advisable to pretest the proposed interview questions against NLP methods as the form of a question affects NLP’s ability to negotiate imprecise responses.
Habicht and colleagues’ approach appears to straddle the two – first performing thematic analysis on a sample of the feedback and then using this in a supervised model. Whilst the authors provide a detailed discussion of this analytical approach, they offer less by way of justification. Do they consider this arm to be qualitative research? Or is it simply that the analysis was performed on ‘qualitative free-text’?
Either way, it seems important to note that aspects of the supervised NLP topic classification was carried out on text with an average entry length of 51 characters. That is roughly the length of this sentence. Whilst it may seem like the question of ‘potential mechanisms’ has been answered, how we ask these questions matters.
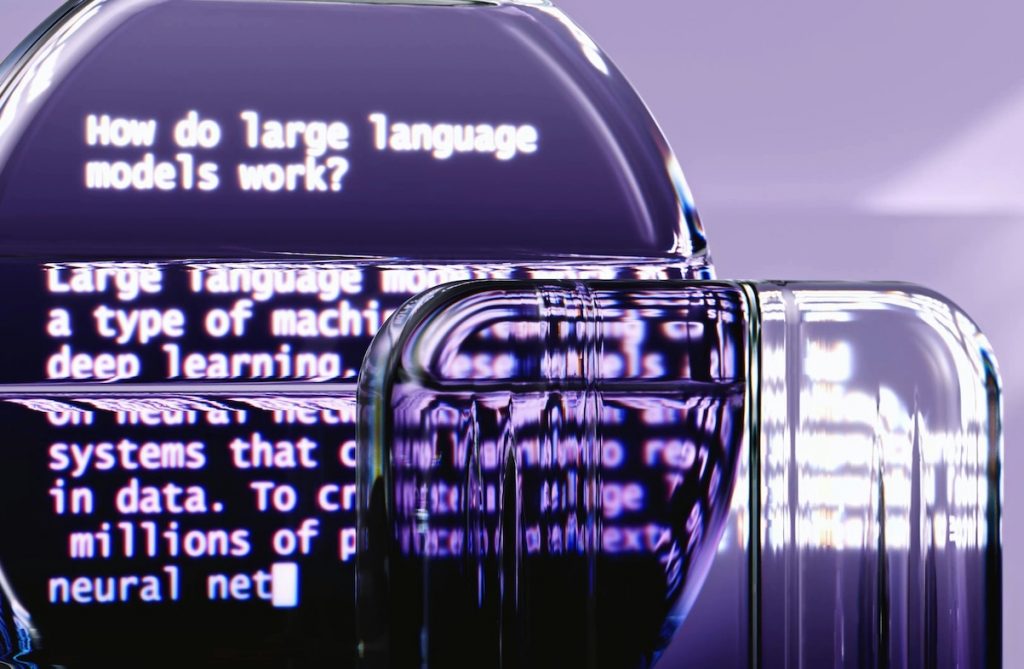
Whilst natural language processing clearly provides insight into the mechanisms underlying these findings, rich qualitative research seems necessary if we are to further unravel these complexities.
Implications for practice
It is here that we can return to the question of ‘where does this all leave us going forward’? Dr Niall Boyce from Wellcome asked a similar question of the article in a recent summary:
An empathetic chatbot is preferable to filling in a form unaided, which is perhaps not the biggest surprise. It’s possible that chatbots can help a more diverse range of people to access services…but what then? Would a “human free” therapist be safe, acceptable, and appealing as people continue their journey?
This is useful in helping frame some initial thoughts on implications.
First, the study does suggest that it is more than simply being preferable to filling in a form unaided. The authors directly compare the personalised self-referral chatbot with a standard webform and an interactive and user-friendly – but not AI-enabled – chatbot. Scores on the user experience questionnaire were higher for the self-referral chatbot than all other forms, but there are some challenges here (e.g., asking participants to imagine themselves in a self-referral situation).
Second, we do need to continue to ask how personalised AI-enabled chatbots can increase self-referrals and why this increase is more pronounced within minority groups. We also need to be mindful- as Andy Bell makes clear in a recent blog on this site – that “mental health is made in communities, and that’s where mental health equality will flourish in the right conditions”. How do chatbots work with and against the importance of communities, for example?
Third, it is interesting to note that the absence of human involvement was seen as a positive by some – especially as the literature appears equivocal on this point. For example, a recent review highlighted how one study found that patients preferred interaction with a chatbot rather than a human for their health care, yet another found that participants report greater rapport with a real expert than with a rule-based chatbot. Somewhat similarly, perceived realism of responses and speed of responses were considered variously as appropriate, too fast and too slow (Abd-Alrazaq et al., 2021). Within our own research on expectations, participants did not view chatbots as ‘human’ and were concerned by the idea that they could have human traits and characteristics. At other points, being like a human was considered in positive terms. The boundaries between being human/non-human and being like a human were not always clear across participant’s narratives, nor was there a stable sense of what was considered desirable.
Part of the reason why both the literature and our own results appear complex is because of heterogeneity in what chatbots are and what they are being used for. Reviews will often include chatbots used across self-management, therapeutic purposes, training, counselling screening and diagnosis. Within our own study, chatbots were being imagined as both a specific and generic technology – for example a chatbot for diagnosis as well as a more general ‘chatbot for mental health’ – leading to a range of traditions, norms and practices being used to construct expectations and understandings (cf. Borup et al., 2006).
This distinction between specific and generic may be helpful when thinking about implications for practice here. Returning to the paper under consideration, Habicht and colleagues do make clear that implications for practice relate to the use of a specific technology – a personalised AI-enabled chatbot solution for self-referral. In this specific instance, the absence of human involvement is seen by some as a positive.
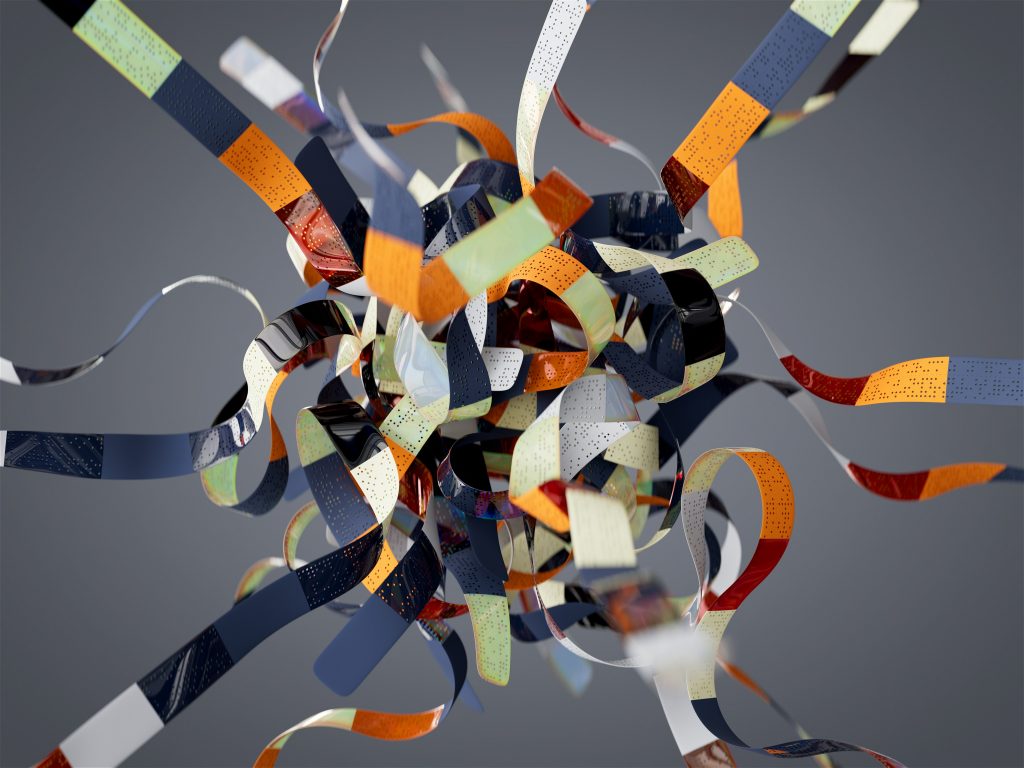
How do chatbots work with and against the importance of communities? This question, among many others, still needs to be addressed.
Statement of interests
Robert Meadows has recently completed a British Academy funded project titled: “Chatbots and the shaping of mental health recovery”. This work was carried out in collaboration with Professor Christine Hine.
Links
Primary paper
Habicht, J., Viswanathan, S., Carrington, B., Hauser, T. U., Harper, R., & Rollwage, M. (2024). Closing the accessibility gap to mental health treatment with a personalized self-referral Chatbot. Nature Medicine, 1-8.
Other references
Abd-Alrazaq, A. A., Alajlani, M., Ali, N., Denecke, K., Bewick, B. M., & Househ, M. (2021). Perceptions and opinions of patients about mental health chatbots: scoping review. Journal of Medical Internet Research, 23(1), e17828.
Bell, A. (2024). Unjust: how inequality and mental health intertwine. The Mental Elf.
Borup, M., Brown, N., Konrad, K., & Van Lente, H. (2006). The sociology of expectations in science and technology. Technology Analysis & Strategic Management, 18(3-4), 285-298.
Boyce, N. (2024). The weekly papers: Going human-free in mental health care; the risks and benefits of legalising cannabis; new thinking about paranoia; higher body temperatures and depression. Thought Formation.
Centre for Mental Health (2020). Mental Health Inequalities Factsheet. https://www.centreformentalhealth.org.uk/publications/mental-health-inequalities-factsheet/
Leeson, W., Resnick, A., Alexander, D., & Rovers, J. (2019). Natural language processing (NLP) in qualitative public health research: a proof of concept study. International Journal of Qualitative Methods, 18.
Lowther-Payne, H. J., Ushakova, A., Beckwith, A., Liberty, C., Edge, R., & Lobban, F. (2023). Understanding inequalities in access to adult mental health services in the UK: a systematic mapping review. BMC Health Services Research, 23(1), 1042.